What VCs and Founders Get Wrong About the Credit Business
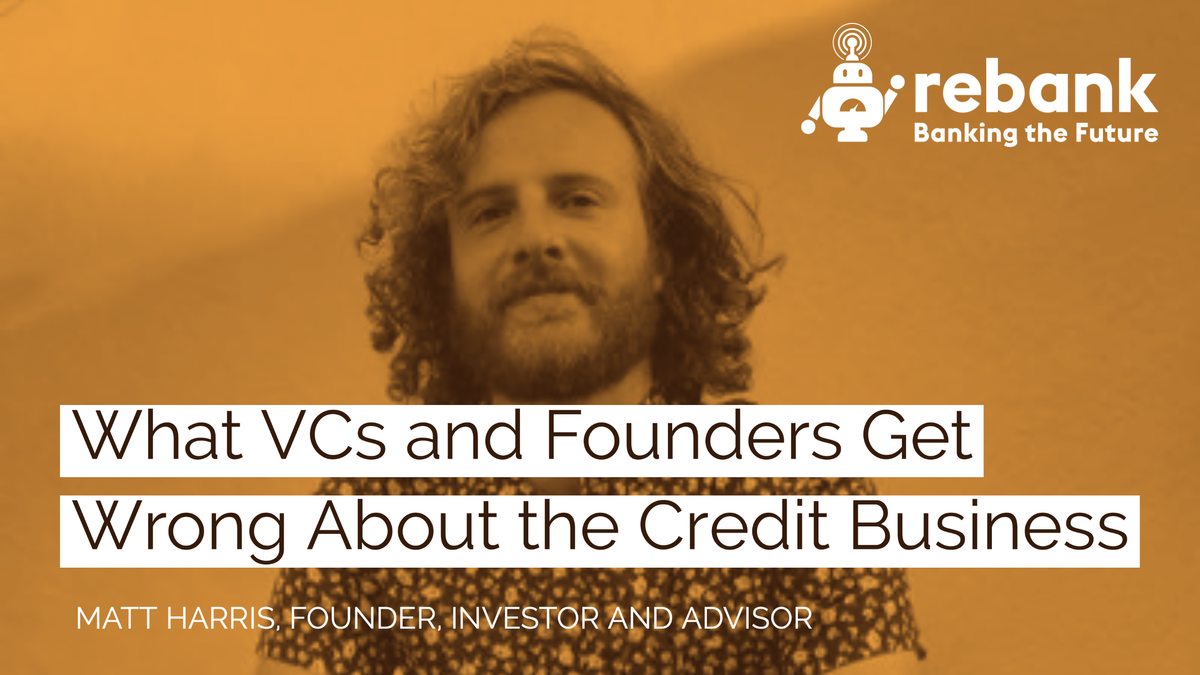
Matt Harris is an entrepreneur and investor and the founder of Bloom Credit, an API platform for credit data access and analysis.
Currently, Matt is focused on investing and advising, working with VC-backed founders and advising companies in the credit space.
Among other things, Matt is an advisor to Commerce Ventures, writing scout checks and helping the fund make fintech investments.
Matt is also an active angel, having invested in 10+ fintech companies including TrueAccord, Karat and HM Bradley and a number of others.
In this conversation, we discuss the level of understanding among VCs and founders of the credit business, the inputs to effective underwriting, how to build a great credit business, emerging opportunities like embedded credit and more.
Key takeaways
Debt investors often have more granular visibility into credit performance than VCs due to the nature of the role, their experience and the depth of their diligence. However, seeing a fintech lender raise a debt facility is not necessarily a strong indicator of the underlying business. Debt facility providers have more protections that equity investors in the event of credit losses, so the investment calculus is different.
Fintech lenders during the last credit cycle commonly raised equity based on growth metrics and aggregated portfolio performance data rather than vintage data. For instance, rather than showing how loans issued in 2019 off a specific $50m credit facility performed as compared to those issued more recently using a different facility, companies blended all loan performance. Aggregating had the effect of diluting the impact of losses on older vintages, giving the impression that performance was better than it actually was. When evaluating investments in fintech lenders, be sure to analyze loan vintage data for more accurate performance visibility.
A lot of lending portfolio data is public and accessible via sources like https://www.kbra.com/.
Like balance sheet banking, lending is not a business well suited to venture scalability expectations. Rapid deployment of capital to borrowers to show growth and drive origination fees will almost inevitably lead to high default rates and enterprise value destruction over the credit cycle.
The best lenders combine behavioral and contextual customer data with financial data to identify great borrowers. More specifically, they seek primary data, meaning data collected directly rather than sourced, around which to build lending businesses. Companies should then start slow, testing a credit product and underwriting hypothesis with a small pool of capital before raising a larger facility and scaling operations.
The best opportunity to build a credit businesses is on top of a naturally adjacent product or service with the goal of increasing LTV (by adding incremental value and extending customer lifetime). Start with the existing customer base and data set and reverse engineer an underwriting model. Take the anonymized data set to the bureaus and get financial profiles, which you can then use to enrich your underwriting, raise debt capital and position your offering in the market.
Extending the above strategy further, think about what new primary data sources you can develop, potentially by new products you can build or acquisitions you can make. Rocket gets great primary data through its ownership of Truebill.
There were two different types of fintech lenders that struggled in this cycle. One group, including Upstart and OnDeck, grew too fast, underwrote loans poorly and ultimately lost money. Upstart’s stock is off 85% over the last twelve months as of March 3, 2023. OnDeck, once valued in public markets at as much as $1.9bn, was sold for $90m in 2020. The other type of lender, exemplified by Bond Street, realized that they couldn’t blitzscale origination to meet growth goals and ultimately wound down responsibly.
Embedded lending is a big opportunity when focused around improving the LTV of existing customers of adjacent products and services.
In Matt’s view, we will see many fintech companies that touch money (e.g. that actually serve financial products to customers) consolidate or go away in the next twelve months. Pure software providers operating in the fintech value chain, for instance API services, KYC/AML, fraud tools and others, will continue to be successful and grow.
Full transcript
Will Beeson:
Matt Harris, welcome to Rebank.
Matt Harris:
Thank you. Glad to be here.
Will Beeson:
Likewise. Really cool to connect with you. So maybe to kick off, a quick intro on you and then we can dive into the meat of the conversation.
Matt Harris:
Yeah, absolutely. Appreciate you having me on. Matt Harris. A lot of people know me as the founder of Bloom Credit. We're an API that helps people more effectively connect with the three credit bureaus, Experian, Equifax, and TransUnion, both for pulling credit data from the three bureaus, but also more effectively furnishing data back to them. I was the CEO of the company for roughly six years and stepped down roughly two years ago, give or take, and have since just been doing a couple of different things, most notably, doing a lot of different investing and advising, working with more than 10 different fintech companies. Some highlights include TrueRecord, Karat, HMBradley, Manai. In addition to that, I also worked as an advisor at Commerce Ventures, where I helped them write some checks how to define, whether they be scout checks in seed companies or some different core investments they do. And then on top of that, I currently work as an executive coach to 10 different founders, all of whom are VC backed, most of whom are in fintech, but all across the board. So that's what I'm up to these days.
Will Beeson:
Awesome. Well, lots to unpack there. I'm sure there are a handful of conversations that we could potentially have. But the one that I think would be most interesting today would be around credit fintech specifically. We've seen a lot of different models over time. You and me now, I think we've been in this long enough to have witnessed more or less a full credit cycle in fintech, which I think cannot be said for probably the vast majority of people working in this space. When did you start Bloom Credit?
Matt Harris:
We started Bloom really in 2016, but had raised money probably end of 2016, early 2017, was when that round closed. So Bloom's been around for roughly seven years now. My first fintech experience was roughly in 2011 at a company called LendKey, where I held a series of roles relating to either product or marketing. And so that was really where I cut my teeth in terms of starting to learn how lending works and things like that. Shamir, one of your previous guests, has so kindly dubbed me the youngest OG in fintech.
Will Beeson:
I can see that. So you've been in credit fintech then for 12 years, more or less. What are your high level takeaways? Or maybe a more specific question, to what degree do venture capitalists and fintechs understand the credit business?
Matt Harris:
Maybe this will be harsh, but almost not at all. It's a business where people have a series of making pretty strong assumptions about how the business models are supposed to work, without actually doing the underlying information behind them. And so we've really seen that play out over this last credit cycle, and there's a bunch of people who say this in and around Twitter, but you can pretty much almost entirely describe it as people not really grasping on a business model level. The idea that giving money away is easy and getting it back is hard. And so we've seen a series of things that have been funded because they were able to grow really quickly but weren't actually good lenders. And we can get into how these companies raise money and really what VCs miss in that regard, but things like alternative data, things of that nature, never really made much sense to a lot of different people who had been in credit for a long period of time.
And so we're finally starting to see now on the back end of the bull market into this bear market, what that really means and why. And you see that play out in some of the performance of some of the public companies like MoneyLion and Upstart, see that play out in the fact that delinquencies and defaults are going up a lot. But there were a lot of things that were pretty avoidable mistakes to people, that even as recently as 2017, companies that wound up going public, debt investors were talking about how they were bad stories. So it's been pretty interesting just having been in the space a while to see debt investors who really look at all these businesses on a unique economic basis versus VCs and founders who go and launch these businesses, having just pretty bifurcated opinions about what was happening in the market. And we're finally starting to see it settle itself in terms of really where a lot of the debt investors saw things going. So I don't know if that answers your question.
Will Beeson:
Yeah, definitely. Debt investors, to clarify terminology, that means people providing credit facilities, warehouse facilities that people are lending on the back of.
Matt Harris:
Yes, correct.
Will Beeson:
Digging in there for just a second, if you're a lending business, one of these perhaps businesses with some sort of fundamental flaw or at least opportunity for improvement, you're raising equity capital from VCs, you're raising debt capital from debt providers. You're saying that there are challenges in understanding how the model works, or again, opportunities for better understanding on the VC side and on the founder side, but the debt providers get it. I guess the debt providers are providing capital to these businesses.
Matt Harris:
And the debt providers get a much faster loop in terms of feedback as to whether or not they make good or bad trades. The debt providers are also a lot more empowered to be able to get off of certain loans if it's not going particularly well and they put a lot more protections in place than a VC can. The most interesting thing I could say in terms of what happened in this last cycle, in terms of the disconnect between the two was really the degree to which VCs did not understand the concept of a loan tape. And I've seen a couple of these decks now, being on the other side of things, what you often end up seeing a lot of startups do when they actually go raise equity money is let, let's say hypothetically a marketplace lender, and what they do is they get paid mostly on origination fees. So they get paid for the attempt of making a loan.
And that can be anywhere between $500 to a thousand. Can be more than that depending on the type of loan you're making, like in mortgage, it can usually be $2,500 to $3,000. But you're getting paid on an origination, so you're not necessarily getting paid more or less based on the performance of the loan, but you need the loans to perform. So what you'll often end up seeing as a company being like, "Cool, we've raised our first debt facility, it was super small," and what we actually go and we do with that is we issue a hundred loans. Now, let's say 10 of those loans defaulted. Now what you can technically do is raise another debt facility. Those terms may not be particularly good. Then go issue a thousand loans and then basically say on aggregate, your default rate is quite low. So what you often see is a slide in these decks that says something to the effect of, look, our loan volume went up but our defaults went down. And then what would happen is you'd ask these people for the loan tape and they disappear,
Will Beeson:
What's the loan tape?
Matt Harris:
Basically what a loan tape is, is when you think of a lending company, you can't think of it in terms of total performance. You have to think of it in terms of vintages. So a first vintage may be that first hundred loans, then there's going to be a second vintage, which is that thousand loans. So really when you look at it in terms of aggregate performance, you can always delete your own loan book. And so what a lot of VC backed companies did, is they've raised larger and larger and larger amounts of equity by basically saying, "Hey, look at our loan performance. It's getting better all the time because we're deleting our own loan book."
Will Beeson:
Because basically, 90% of their loans they issued within the past three to six months. So they haven't even necessarily had time play out, is what you're saying?
Matt Harris:
You have no idea how those loans are going to perform over a long enough period of time. But VCs were looking at these aggregate numbers. It's not that the companies were lying, it's that a lot of the investors just didn't understand what they were getting into. So an example of this that I talked about a lot, I've talked about publicly before, is Upstart. Upstart performs with a genuinely negative vintage. So like an average vintage, if you're looking at any type of lender, should be somewhere between five and 8%. If upstart lends at a hundred dollars, they're going to lose one and a half dollars. So get back $98,50 on average based on vintage data that I looked at probably a year ago. I'm sure that's changed over some period of time. If anything, it's probably gotten worse. But 2021 vintages and 2022 vintages look roughly like this.
And so this data was true in 2017. So a lot of times, what we would see, is it's like it's pretty clear on paper that that business is at some point going to run out of the ability to raise debt capital, but they can continue to raise, they keep actually triggering their early net loss and amortization rates, which is a means of saying the lender can start collecting more, as the origination for every net new loan if the loan performance isn't particularly good, to make up their loss. And what would end up happening there is that Upstart would go raise another debt facility, they'd issue a bunch of loans, the loans wouldn't perform well, they'd raise another debt facility on worse terms and worse terms and worse terms, to the point where they couldn't do that.
And so where this comes to a head is Jim Kramer calling out Dave Gerard on CNBC and being like, "What is going on? Why are you balance sheeting these loans? This wasn't the point." And the truth is because Dave Gerard can't raise money from anyone else at that particular moment in time. So this is a long roundabout way of saying, these things take a really long time to play out. And if you're only measuring them on a year-to-year basis when they're between raising their series A, series B, series C, on the performance of ability to give loans away, then you miss the other back half of it. And so there's a bunch of businesses that wind up having really bad outcomes in the public markets, probably most famously would be OnDeck. And this is exactly what was happening to OnDeck. In reality, nothing about the lending business is actually very valuable. And so a business that at one point was multiple billions of dollars in enterprise value, winds up being sold for less than a hundred.
Will Beeson:
So let's dig into there. Imagine that you are in your day job advising a venture capitalist. So they're either looking to make an investment in a company like this or they have an investment in a company like this. What advice are you giving to them and also to the founders, operators of the business in terms of how to run a good credit business?
Matt Harris:
And by the way, one thing I want to name is that these things take years to figure out. It wasn't like I woke up one day and was like, "Oh, I totally understand this". It's a really complicated business that has a lot of issues that are going in and out of it in terms of cost of capital, in terms of even the concept of, I don't think most VCs know that most lending vintages are public in some capacity. How would you know that if no one ever told you? So often with times when I have those conversations with the VCs, it's the first time they're figuring out they can look up Upstarts performance on kbra.com. And so there's definitely a lot of that. But what I often talk about with companies that are launching credit products, is this idea that you want to build the idea of the lending business into your business model.
And so when you look at the best performing lenders, oftentimes what they look like is that they're doing something that is core to the nature of the business model that then the lending actual aspect can be a LTV boost on almost. So a really good example of this is, despite the fact that many non-bank lenders haven't performed in this cycle, it's worth noting that Rocket Companies does a very good job at performing as a non-bank lender. They basically have two funnels for the vast majority of their products. The first one is mortgage, and the second one is the acquisition they made in Truebill. And so the idea is, "Can we see you perform in other products that are not risky?" In other words, "Can you actually manage your subscriptions? Can you actually pay a mortgage back?" And that gives us data that gives us confidence that we can give you other types of loans.
And so for example, let's say that you're a company that runs an investing app or something like that, or you're a business that helps people more effectively manage their cash flow or their subscriptions, you'd actually want to take primary data of your best possible users and reverse engineer an underwriting model from there. What most people try to do is they're like, "We're going to find a crazy data point that provides some alpha like, SAT scores, but there's no way to actually verify whether or not those consumers are going to be better or worse. So what ends up happening is they take these really big risks, they can't necessarily change the mid-flight of the company and then they're stuck trying to figure these things out. And so the thing that I always talk about is that the best alternative data is the one that actually comes from your existing business model, and that's stuff that's going to be found in your actual user level data.
Can you find the best possible users who are showing positive selection and then basically reverse engineer their underwriting criteria from there, rather than attempting to guess their underwriting criteria and finding out that you guessed incorrectly after you've done two to three years of lending products?
Will Beeson:
In your mind, what are the pros and cons of just a traditional FICO score based underwriting model versus the reverse engineered... I don't know if alternative data or alternative credit scoring model is the right term.
Matt Harris:
I think the thing about FICO that tends to get lost in this debate, is that most people think of FICO is an underwriting tool. And again, this is part of what makes it so complex is that FICO is actually not a very effective underwriting tool on its own. So in some sense, the VC narrative has been correct for a long period of time. If you were just to throw FICO scores at people, that's not necessarily what you want to use them for. FICO is a securitization tool and so it gives you a good understanding of cohort performance versus individual performance. So oftentimes what we tend to have a conversation as, it's an either or. In reality, it's both. You want to actually know what those FICO scores are as a means of more properly, A, securitizing your loan and getting better cost of capital because you want to have people with higher FICO scores, but also simultaneously because you're trying to underwrite people on a cohort basis.
The thing that FICO provides that most people don't understand, is that FICO provides incredibly valuable orthogonal data, and what that means is that I can see how FICO performed in the 2000 dot-com crash, in every single financial crisis leading up to this day and age that allows me to more effectively understand how to underwrite a consumer in financial distress. And so it's a good basis in a starting point for an underwriting model, A, because it helps you securitize and B, because it gives you that level of historical understanding in a manner that other types of data points won't have. And so oftentimes it's as I mentioned, we frame it as an either or and really the way we want to think about it is we want to use FICO, but we also want to understand our customers more effectively. And really when we're talking about this idea of alternative data or anything like that, what we're really discussing is contextual data.
Do I understand why this consumer does what they do in context? There's a story I like to tell, which is a really good means of understanding this. When Bloom raised it's Series A, I had two banks, they did a financial action with me both on the same day. As a founder I'd been running my credit limits relatively close to the vest for years. And so basically we raised our Series A and I started to make some payments'
Will Beeson:
Personal or company?
Matt Harris:
Personal payments. And what I see is that Chase and Bank of America moved my credit limits backwards. So as soon as they see that I can make a payment, they're like, "Oh, sorry, actually we're going to limit you to what you already have." So they're basically saying, "Hey, we're not actually going to keep letting you borrow money the way that we were going to before." On the same exact day, Goldman Sachs reaches out to me and wants to be my private wealth manager and wants to start managing my assets. The important point is that JP Morgan and Bank of America are looking at me on a cohort basis. They're like, "People who generally look like you don't really figure it out." Technically correct, no disagreement with them. When it happened, I was like, "Yeah, that makes sense." Simultaneously, Goldman actually understands the context, because they know who I am and they know where it came from and they can track me personally.
Will Beeson:
And how do they have access to that data? They read TechCrunch or they had your checking account or something?
Matt Harris:
They understand the concept that a founder might actually make those decisions, that that's not indicative of my financial future, and that as a result they still want to work with me, whereas another bank is trying to basically get rid of me. And this happens all the time, which is why when we talk about alternative data... Stuff in a vacuum is not that interesting, it's what can you know about your customer on a primary basis? And we see this play out in the underwriting data over and over and over again.
Will Beeson:
And you think that that was like a relationship manager type person doing manual work or that was some data platform that Goldman has to help them?
Matt Harris:
I'm sure there's a bit of both. In terms of how they figured it out, it's not hard information to find. It's just when you're looking for ones of one, you can do less scalable things versus when you're trying to underwrite tons of people, but there are companies that do that scalably also. A lot of people who know me also know I'll bring up Opportune a lot and it's because Opportune is the best underwriter in the country, lending to a average 638 FICO score and it's because when they want to give you a loan, they have you walk into a branch and they talk to you. It's actually not that difficult to get the type of data you need to understand the context of why someone's financial life is the way it is. The issue is that all tech companies attempted to be really scalable in this regard.
And lending is more of a push and pull. You want to be scalable because you want to issue a lot of loans, not create some level of friction, but also simultaneously you need to have enough friction in the process to actually add some level of positive selection for people who are going to be diligent in their application, while also simultaneously learning enough about them that you can understand the context of their life. There's a bunch of nuances to how these things work, but that tends to be the case.
Will Beeson:
Okay, so you talked about FICO score, you talked about contextual data. If you're thinking about designing from scratch, a credit underwriting model, what are the core components that you want in that model and how will you get them?
Matt Harris:
I think the first thing you have to ask yourself is, "What am I attempting to accomplish with this lending product" is it meant to be its own standalone P&L, because I'm trying to launch a net new business on top of this? Am I using it to acquire new customers I don't currently have? Am I trying to boost LTV on existing consumers? What are the things that are going on there? The thing is that money is so commoditized that oftentimes companies try to just be like, "We're going to be a better brand with a better user experience in terms of helping people get access to a loan," but that only takes you so far.
In reality, the major banks have really good user experiences and also simultaneously have much better brand recognition. And so what we tend to see is when companies try to go and just be like, "Yeah, I'm going to launch a new business model and there's going to be a whole new P&L that we're launching on top of this, and they think about it from an overall, "How do we move horizontally perspective," they tend not to do very well because the models tend to be equity inefficient and that the larger and larger and larger their lending programs get, the less effective they are over time on a unique economics basis. I think this is true of a lot of consumer fintech at this point.
Will Beeson:
And it's mainly customer acquisition cost or it's that they're lending increasingly risky and therefore losing capital.
Matt Harris:
Can depend on the context. It often is, customer acquisition cost goes up as you get larger and larger and larger, you have to compete with the major banks more. And so they're big brands that are very recognizable and so the result is it's like thinking about, if you walk into a store for the first time, you're trying to buy two identical pairs of shoes, are you buying Nike's or GREATS? GREATS was run by a friend of mine, so, great company, but it's like the truth is GREATS won't do well in a head-to-head against Nikes over and over and over again against the general population. So that's one example where they've become equity inefficient. The second example is that you actually need to take on more cost structure to keep managing the loan process. So one thing that say, credit cards for example, or even debit cards that people don't get, is that that's a supply chain problem.
You literally need to ensure that you are constantly getting plastic in the hands of consumers or metal or whatever it is you're doing. So the larger and larger and larger it gets, we tend to not see economies of scale going into those particular processes because it's, A, a supply chain problem. Also, the cost of servicing these people gets a lot higher because you're dealing with more and more issues, which means you need to hire up more operational people as a result of it. So there's a bunch of ways that these businesses tend to become equity inefficient. What I think we tend to see work in terms of lending models, is two things, and actually really they boil down to the same thing, but it's really when you're looking at how do you boost LTV more effectively.
Can you be selective in the type of people you want to work with that are going to actually allow you to build a profitable lending business because you're attempting to be like, "Look, we already have this relationship with you, it's really good. Can we give you something preferred that you're not going to get elsewhere? That allows us to actually continue to develop a deeper and deeper relationship with you." And it might only be with your top five to 10% of customers, but it's, "How do I really capture and own this consumer," as the idea, rather than the idea of, "I need to launch a new business line."
So you may have a credit card, but it may not be that high. So the first thing I always tell someone to look at is, "Don't try to look at the financial data." Everyone tries to go to the financial data first. That can be valuable, but the thing is it tends to narrow our scope of who we can work with. If you look at Opportune versus Upstart as the example of this, Upstart lends to nearly a 700 FICO, Opportune lends to a 640. That's a massive difference in terms of credit quality on a cohort basis. Yet somehow Opportune can produce a nearly 20% vintage yield and Upstarts are generally anywhere between negative one and a half and 2% depending on the vintage. So the credit quality and the actual financial data is not necessarily the most important thing. What Opportune is doing is they're getting contextual data.
So we look at who are the customers that we think are our best possible customers, how do we boost LTV? And from that point you can generally see their actual performance in terms of who are these people, what are they doing on our platform and what are the behaviors we look for in terms of positive and negative selection. So to simplify, the first thing is behavior, and then the second is categorization of behavior. And so when we talk positive and negative selection, we talk about the degree to which we see types of behavior that tell us that someone is likely to more effectively repay a loan.
One company that's pretty public about their underwriting model will be Tala, which is interesting to talk about in that Tala is based overseas, but if you look at what Tala underwrites on someone who does have infinite ability to underwrite our alternative data because they're not based in the US, Tala tends to look for stuff like, "How is your contact list formatted?" First name, last name means you are a positive selection for good lending quality because you are diligent in the manner in which you organize information. Another example would be, "Does your phone stay charged throughout the day?" If your phone is generally going down and then getting recharged again, says there's something consistent about your behavior versus people who are constantly going between 20%, 40% back to 15%, up to 25%, so you don't have consistent access to actually going into the same places at the same time. Tala will also look at stuff like, "Do you consistently commute into the same places? Are you going to and from work every day or are you going to different places every day?"
Diligence of behavior, consistency, these are the things we look for in underwriting versus stuff that's negative selection, can be all over the place, but as we just called out, things that show lack of diligence in behavior. So an example that we tend to see in US underwriting applications would be literally the amount of characters and punctuation you put in your job. If we were to say, Matt Harris is applying for a loan, if I wrote that CEO in all lowercase, I'm going to be significantly worse performer than chief executive officer with the C, the E and the O capitalized. And so we're typically looking for a lot of stuff like that and so we want to find where that exists in our existing customer base and from that point onward, what we really want to do is we want to start with a small lending cohort, because we want to test our hypothesis.
It's like, "Hey, this is the type of loan program we want. We want an LTV boosting loan program. They're going to be a series of these things, but we'll just go with that for now." And then the next thing we say is we say, "Cool, people who use our service, let's say, people who can typically save a hundred dollars using Mint are going to be good performers from this because they're actively paying attention to their finances and they're willing to take action to improve it." Now what we can do from that point is actually take that data, go to a credit bureau and be like, "Hey, here's the anonymized information of all these consumers. Give me the data of what their financial profiles look like." And you can start then being like, "Okay, cool. Our average FICO of people who take this action is 680, blah blah, blah, blah."
This gives you the data points that you need to go get a debt facility. Start small, it's going to be relatively expensive the first time you do it, but verify your hypothesis. You're going to be happy you did it because when you actually start small, do a small version of your lending program and it performs, you're going to be able to raise at much better rates when you actually need to go again and again and again back to debt capital providers. And that's going to ultimately be what allows you to boost margins in LTV is the ability to go slow and steady. Now, most people don't want to do that and that's not really the venture capital model, but again, we've seen a ton of examples that non-bank lending can work. There's a narrative going around that's like, "Yeah, lending sucks not going to work."
And it's like, "Well, it can. The problem is it doesn't fit the way that VCs want companies to build." You can't build a lending business at light speed, it just won't happen. You are so likely to make mistakes, you're likely to miss learnings and you're probably going to take a lot of unnecessary risk attempting to grow faster than you need to. It's not what I think VCs want to hear, but it's this idea of that you can ultimately do it, you just need to be willing to be a little bit more slow and sustainable over that period of time. So that's how I see it, in that that's a formula that can be repeated, but it's highly contextual to your customer base and where can you find that user-based data and be clear about what your goals are with it.
Will Beeson:
If we pull the two pieces together, the exchange of the beginning around the shortcomings of some of the higher profile alternative lenders or fintech lenders that we've seen, and the approach that you just described, is it as simple as the companies that didn't do a good job, were just trying to go too fast, trying to grow too fast, trying to get loans out the door, or do you think there was something different about it? Because I assume at the end of the day everyone has credit people that on some level know how lending works, know what inputs into a good underwriting process are. Lending has been a bread and butter business for centuries.
Matt Harris:
Yeah, totally. I often describe this as, if you think of the history of venture capital, venture capital has up until very recently, almost entirely funded deep technology companies. We're talking like Genentech, things of that nature that were happening in the sixties and seventies, Apple computer in the eighties. These are technology companies first and foremost that need a lot of upfront investment and then once they get it, they find equity efficiency. What we found in this last cycle was lending does not work that way because lending is a commodity. So once you actually can get the loan off the ground, there's nothing sneaky or proprietary about it. And what VC has really tried to do was think of the idea that the data analysis was going to be the proprietary thing that was going to lead to the equity efficiency. The thing is that what most people don't understand, who aren't technologists, is that hypothetically speaking, we could create a model that dictates your and I credit performance, based on the nature of this conversation, and it'll give you an answer.
Just because it has an answer doesn't mean it's a correct answer. So basically everyone heard this concept of more data equals better insights, turned it into, "Our algorithm is going to be vastly superior," and the truth is, underwriting cost of capital, all these different things are commoditized. And so attempting to run these businesses like they were classic venture capital businesses, didn't make sense. Now that's not to say that there isn't versions of that that exists. One business that I think is doing something really cool right now is Yendo, which really started with title lending, but what they were really doing was this concept of like, "Okay, cool, we're going to go do a secured loan, we're going to see how people do on the secured loan, gets us better data than we can go expand into other types of things." It just takes a little longer. So the go, go, go, break-things-at-all-costs thing and lending didn't really make sense because the nature of the business model in lending doesn't lend itself very well to being a break-things at-all-costs type of things.
So it's why you've seen companies like Rocket be structurally exactly the same, maybe not exactly the same, I don't want to be that technical, but very similar in structure to a MoneyLion, to a Upstart, to a name your favorite fintech lender, and yet be so incredibly successful and have such a large enterprise value versus the other. But they also grew over a 30-year period. Structurally they're not that much different. They don't have a banking charter. They have to go raise debt facilities for a lot of the stuff they do, but they manage to do it profitably because they take it slow, steady and they iterate. So that's the thing. I think to more succinctly answer your question, what we tend to define was there were two different types of failures of companies that were in this lending business in the last cycle.
Nothing against any of them, and actually have a lot of respect for a lot of the founders of the ones I'll even name, but I think we tend to define two problems. One, is what I call the Upstart problem we'll say, because I've mentioned them a couple of times. You're going to grow at all costs. You're going to actually chase the origination volume and what's going to end up happening is you're going to have a reckoning as you continue to raise more equity that keeps the business moving, you're going to raise worse terms at debt facilities, to the point where at some point the business model just doesn't make sense because you're losing so much money on every individual loan that it's just a matter of time before it crashes. So we had a bunch of those. OnDeck is an example of that, Kabbage is an example of that, where the business model can't sustain themselves over a long enough period of time because the nature of the performance and the nature of the means by which the business does revenue, are not in alignment.
The second one you have is what I would call the Bond Street, which is basically you actually are really focused on credit performance, but you can't find enough loans to continue to justify the equity raise, which is why those guys ended up making a decision to be like, "Hey, hypothetically we could go this other route and we could just start praying and praying and seeing what happens and continue to raise equity money," but they chose to wind it down at their Series A, joined Goldman, basically upon realizing that that was the case. And so David Haber is super smart, as is Eddie and a lot of the people who are involved in that business, and so you've seen them be really successful in other capacities. David now being a partner at a16z, Eddie running the Stripe Capital business, and so they've learned a lot of these lessons, but those were really the two bifurcated examples.
Is there some nuance for every single business? Yes, absolutely. We really found that that was the case, and so now we're seeing, on the other end of it, having been through the credit cycle, people get more creative and do some stuff like what Yendo is doing.or another example of a company that does an embedded lending type of product is O5, who really are enabling payments platforms to more effectively give working capital solutions. But rather than being a full-on lender and being like, "Hey, we're going to go give working capital solutions directly, they find ways to partner with people through these other different types of services they're going to use anyway. We're seeing this next generation be a little bit more clever about the means by which they grow rather than just being like, "We're a lender with some great design, a really nice web two name and some sweet algorithms.
Will Beeson:
You mentioned embedded lending. That's exactly where I wanted to go next. At the high level, the concept of embedded credit, how much does that excite you?
Matt Harris:
I think it does. I mean ultimately it's all contextual. There are certain versions of it that make a lot of sense to me and there's certain versions that don't make very much sense at all. It comes back to this idea of, really is the nature of what you're doing the force multiplier for the underlying technology behind it, in that lending, when done properly, is a means by which you should ultimately be able to capture and have a stickier customer base over time. And so having the ability to have these embedded lenders actually go, and in the example of O5, be more effective for these payment providers to have more effective merchant solutions, makes a ton of sense to me. Those are the types of things where I think it makes a lot of sense. I think what we're learning in terms of what ultimately makes less sense, is that you're seeing also a bunch of different scenarios of people trying to be like, "Hey, we're going to be embedded by just having a series of partnerships with merchants and just doing different variations of buy now, pay later."
Now, I've historically been a pretty big fan of buy now pay later, in terms of its ability to be an enabler of access as a business model. I think what we've found is that as those companies have gotten larger and larger and larger, and also due to the lack of reporting in BMPL as well, which is a whole separate conversation, that a company that's embedded in that capacity or those types of companies haven't done particularly well. I guess to put it more succinctly, you can be a lender. Are you a force multiplier as a lender or are you ultimately enabling bad behavior as the lender by being embedded in something like Peloton? Are you just enabling a bunch of people who really shouldn't be buying Pelotons to actually go do so? And I think that's why a lot of those companies haven't necessarily performed very well in terms of their actual credit performance.
What I think we'll begin to see is that as people get more and more into embedded lending, it'll start to look a lot more like Dealertrack, which is not a company that I suspect most people listening to this will know. I don't know when Dealertrack IPO'd. It had to have been nearly 20 years ago at this point. But what Dealertrack basically does, is let's say I'm a consumer, I go into a used auto dealer and I'm like, "Hey, I want this car." The used auto dealer can basically take my application, ping it out to 50 lenders who are willing to give me an auto loan, get the best offer, come back to me. And so whereas LendingTree does this direct to consumer, I think you'll see a lot more of this in a platform oriented basis where I don't even really need to be provider of capital in reality. There's already so much capital out there for consumers.
We can talk about how that will change over the next credit cycle, but there's a lot of capital out there for consumers. There's a lot of merchants and different types of embedded places where they can actually go and get distribution. The thing is these groups don't typically work well together, so as you bring in more middle men to actually make that possible, I think you'll see a lot more of that. And so Dealertrack is one great example and really what is old is new again. We might see some other things that look like that that are echoes of it. That's really where I think embedded will start to go, is this idea of that, "I'm embedded as a technology provider," and really we're all of fintech is going to start going.
We have about 12 more months before we start really seeing the consolidation happen. A lot of these companies have runway, but candidly don't have that much more left. So the consolidation's about to happen. Most of these direct-to-consumer companies are going to have bought for pennies on the dollar, and what we're going to find is standing of software providers and it's because a lot of them are also able to get outside of being directly within fintech. If you look at Sochor as an example Alwar, different groups like that, they sold to a lot of non fintechs and in fact, one of Sochor's biggest verticals is gaming now, DraftKings, places like that. And so you'll see a lot more of that and I think stuff that looks embedded is going to be a lot more software solution than it is going to be an actual finance solution, which is really getting back to the roots of what venture capital is.
So in a long story short, the pros and cons of being in embedded lender are really contextual to what you're attempting to enable. There are good versions, there are bad versions. It should look a lot more like software over time, and that's ultimately what's going to be the successful versions is where you're moving money, more so than you are, "I'm actually holding someone else's money on some sort of term and I have to basically act like a bank and have the cost structure of a bank, but none of the upside of a bank."
Will Beeson:
Awesome. Well, Matt, I'm so grateful for your time and insight here. I'm wildly impressed with the breadth and depth with which you can discuss credit. Very, very clear that you spend a lot of time in the weeds here, and I'm sure you provide tremendous value to all the companies that you work with in that space.
Matt Harris:
Thanks, man, I appreciate that.
Will Beeson:
Matt Harris, thank you very much for joining us today.